"Return Rate Reduction: How AI Helps Identify and Resolve Product Issues Before They Scale"
Explore how Artificial Intelligence reduces return rates by identifying product issues early and enhancing quality.
Artificial Intelligence is changing the way businesses tackle product issues before they escalate. With the ability to analyze data faster and more accurately than humans, AI can spot patterns and predict problems that might lead to higher return rates. This article explores how companies can use AI to catch these issues early, improve quality control, analyze customer feedback, and ultimately reduce returns. Let's dive into the various ways AI is making a difference in product management.
Key Takeaways
- AI can quickly identify product failure trends, allowing for timely interventions.
- Predictive analytics help businesses forecast potential issues before they affect customers.
- Real-time monitoring systems provide ongoing insights into product performance.
- Customer feedback analysis through AI helps identify common complaints and areas for improvement.
- Integrating AI across the supply chain can optimize inventory and enhance supplier relationships.
Leveraging Artificial Intelligence For Early Issue Detection
AI is changing how we spot problems with products before they cause a wave of returns. It's not just about finding issues faster; it's about understanding why they happen in the first place. This section explores how AI helps catch those early warning signs, so you can fix things before they become big headaches. We're talking about using smart tech to keep customers happy and reduce waste. It's a win-win.
Understanding Product Failure Patterns
AI can sift through tons of data to find patterns we'd never see on our own. It's like having a super-powered detective for your products. By looking at things like manufacturing data, customer reviews, and even social media chatter, AI can pinpoint exactly where products are most likely to fail. This helps you focus your efforts where they matter most.
Utilizing Predictive Analytics
Predictive analytics uses AI to forecast potential problems. It's not just about looking at what has happened, but what will happen. This means you can take action before issues even arise. For example, if the system detects a pattern suggesting a component is likely to fail, you can adjust the manufacturing process or switch suppliers. This proactive approach can save you a lot of money and hassle in the long run. Anthropic has a Responsible Scaling Policy to address AI risks.
Real-Time Monitoring Systems
Real-time monitoring systems use sensors and AI to keep an eye on products as they're being made and used. This allows you to catch problems as they happen, not after hundreds of units have already shipped. For example, in food manufacturing, AI-powered imaging can enhance quality inspection AI-powered imaging, ensuring food safety. This immediate feedback loop is invaluable for maintaining quality and preventing returns.
Imagine a scenario where a batch of smartphones is being manufactured. Real-time monitoring systems, powered by AI, detect a slight deviation in the screen adhesive application process. Instead of waiting for customer complaints, the system flags the issue immediately, allowing the manufacturer to correct the process before any faulty phones leave the factory. This proactive intervention not only prevents returns but also safeguards the brand's reputation.
Enhancing Quality Control Through AI Insights

AI is changing how we handle quality control. It's not just about finding defects anymore; it's about preventing them in the first place. By using AI, companies can get a much clearer picture of what's going wrong and why, leading to better products and happier customers. It's a big shift, and it's happening fast.
Automated Quality Assessments
Imagine a world where every product is checked with the same level of scrutiny, without human error. That's what AI brings to the table. AI-powered systems can analyze products at every stage of production, spotting issues that humans might miss. This means fewer defects make it to the customer, and that's a win for everyone. Think of it like having a super-attentive quality inspector that never gets tired or distracted. For example, an AI-Powered Automated Product pipeline can be built for Shopify.
Data-Driven Decision Making
No more guessing games. AI gives us the data we need to make smart choices about quality control. By looking at trends and patterns, we can see where problems are likely to occur and take steps to fix them before they even happen. It's all about using information to be proactive instead of reactive.
Feedback Loop Integration
AI can help close the loop between customer feedback and product development. By analyzing what customers are saying about a product, companies can quickly identify issues and make changes to improve quality. It's like having a direct line to your customers, so you always know what they think. This constant feedback helps to improve quality assurance initiatives for CX & ROI.
AI is not just a tool; it's a partner in quality control. It helps us understand our products better, make smarter decisions, and ultimately deliver a better experience to our customers. It's about using technology to build trust and create lasting relationships.
Streamlining Customer Feedback Analysis With AI
Customer feedback is a goldmine, but sifting through it can feel like searching for a needle in a haystack. AI changes that. It's not just about collecting data; it's about understanding what customers are really saying and using that knowledge to improve products and reduce returns. Let's look at how AI makes this possible.
Sentiment Analysis Techniques
AI-powered sentiment analysis goes way beyond simple positive or negative ratings. It can detect nuances in language, understand sarcasm, and identify the specific emotions driving customer opinions. This allows businesses to get a much more granular view of customer satisfaction. For example, a customer might say, "The product is okay, but the instructions were terrible." Sentiment analysis can flag the negative sentiment around the instructions, even if the overall sentiment is neutral. This helps pinpoint areas needing immediate attention. This is a great way to use AI customer feedback analysis.
Identifying Common Complaints
AI can automatically identify recurring themes and patterns in customer feedback, whether it's from surveys, reviews, or social media. Instead of manually tagging and categorizing feedback, AI can cluster similar comments together, revealing the most frequent complaints. Imagine you're getting tons of feedback about a new feature being confusing. AI can quickly surface this issue, allowing you to prioritize fixing it. This proactive approach prevents minor issues from snowballing into major problems.
Improving Product Design Based on Feedback
AI doesn't just identify problems; it can also suggest solutions. By analyzing customer feedback alongside product data, AI can uncover correlations between design choices and customer satisfaction. For instance, if customers consistently complain about a product being too heavy, AI can suggest alternative materials or design modifications to reduce weight. This data-driven approach to product design ensures that improvements are aligned with customer needs and preferences.
AI-driven feedback analysis isn't just about fixing problems; it's about creating a continuous feedback loop that drives ongoing product improvement. By listening to customers and responding to their needs, businesses can build better products, increase customer loyalty, and ultimately reduce return rates.
Here's a simple example of how AI might categorize feedback:
Reducing Returns Through Proactive Problem Solving
It's no secret that product returns can really eat into profits. But what if you could get ahead of the curve and fix problems before they lead to returns? That's where proactive problem-solving comes in, and AI is making it easier than ever.
Root Cause Analysis
AI can dig deep to find the real reasons behind product failures. Instead of just seeing that a product was returned, AI algorithms can analyze data from various sources – customer reviews, sensor data, manufacturing logs – to pinpoint the exact cause. For example, if a batch of blenders is consistently returned due to motor failure, AI can trace it back to a specific manufacturing defect or a design flaw. This level of detail is something that traditional methods often miss.
Implementing AI-Driven Solutions
Once you know the root cause, AI can help you implement solutions. This isn't just about fixing the immediate problem; it's about preventing it from happening again. AI can optimize manufacturing processes, suggest design improvements, and even personalize user manuals to address common points of confusion. For instance, if AI identifies that many users struggle with a particular feature, it can trigger the creation of a more intuitive tutorial or a redesigned interface.
Case Studies of Successful Interventions
Let's look at some real-world examples. A clothing retailer used AI to analyze customer feedback and discovered that a certain line of jeans had inconsistent sizing. By adjusting their manufacturing process based on AI's insights, they reduced returns for that product by 30%. Another company used AI to monitor sensor data from their smart home devices and identified a software bug that was causing devices to malfunction. They pushed out a software update, preventing a potential wave of returns and negative reviews.
Proactive problem-solving isn't just about fixing things; it's about building better products and creating happier customers. By using AI to identify and address issues early on, companies can significantly reduce returns and improve their bottom line.
Here's a simple table illustrating the impact of proactive AI interventions:
The Role of Machine Learning in Product Development
Machine learning (ML) is changing how products are developed. It's not just about adding fancy features; it's about fundamentally rethinking the entire process, from initial design to ongoing improvement. Let's take a look at how ML is making a difference.
Training Models on Historical Data
ML models need data, and lots of it. In product development, this often means feeding models historical data on product performance, customer feedback, and even manufacturing processes. The goal is to teach the model to recognize patterns and predict future outcomes. For example, a model trained on past product failures can help identify potential design flaws before they lead to costly recalls. This is where AI/ML product management comes in, ensuring the models are effectively integrated.
Simulating Product Performance
Imagine being able to test a product's performance in various conditions before it even exists. ML makes this possible through simulations. By training models on data related to material properties, environmental factors, and user behavior, developers can create virtual prototypes and run them through countless scenarios. This helps identify weaknesses and optimize designs early on, saving time and resources.
Iterative Design Improvements
ML isn't a one-time thing; it's an ongoing process. As products are used and generate new data, ML models can be retrained to improve their accuracy and provide even more insights. This creates a feedback loop where product design is constantly evolving based on real-world performance. Think of it as a continuous cycle of learning and improvement.
This iterative approach allows for rapid prototyping and testing, enabling companies to bring better products to market faster. It also allows for personalized product experiences, as ML models can adapt to individual user preferences and needs.
Here's a simple example of how ML can improve product design:
- Step 1: Collect data on customer usage patterns.
- Step 2: Train an ML model to identify areas of the product that are underutilized or causing frustration.
- Step 3: Redesign those areas based on the model's insights.
- Step 4: Release the updated product and collect more data to further refine the design.
Integrating AI Across Supply Chain Management

AI isn't just for fancy gadgets or predicting the next viral trend; it's also revolutionizing how products get from the factory to your doorstep. It's about making the whole process smarter, faster, and more efficient. Think of it as giving your supply chain a brain boost.
Optimizing Inventory Levels
Imagine never running out of your favorite snack at the store, or a crucial part being unavailable when your car needs repair. AI helps prevent these scenarios by accurately predicting demand. Instead of relying on gut feelings or simple historical data, AI algorithms analyze tons of information – weather patterns, social media trends, economic indicators – to forecast what customers will want and when. This means businesses can keep just the right amount of stock on hand, reducing waste and saving money. It's like having a crystal ball for inventory management.
Predicting Demand Fluctuations
Demand isn't constant; it ebbs and flows like the tide. Seasonal changes, promotions, even a viral tweet can send demand soaring or plummeting. AI can spot these fluctuations early, allowing businesses to adjust their production and distribution plans accordingly. For example, a clothing retailer might use AI to predict a surge in demand for winter coats after an unusually cold snap, ensuring they have enough coats in stock to meet customer needs. This proactive approach minimizes lost sales and keeps customers happy. AI-driven forecasting tools enhance supply chain sustainability and resilience.
Enhancing Supplier Relationships
Supplier relationships are key to a smooth supply chain. AI can help businesses choose the best suppliers, negotiate better deals, and monitor supplier performance. By analyzing data on supplier reliability, quality, and pricing, AI can identify potential risks and opportunities. This allows businesses to build stronger, more collaborative relationships with their suppliers, leading to improved efficiency and reduced costs. It's about turning supplier relationships from transactional to strategic.
Future Trends in Artificial Intelligence for Retail
The retail sector is on the cusp of some pretty big changes thanks to AI. It's not just about chatbots anymore; we're talking about completely reshaping how stores operate and how customers shop. Here's a peek at what's coming:
Emerging Technologies
We're seeing some cool stuff pop up. Think about computer vision that can track inventory in real-time, or robots that help customers find what they need. One of the biggest things is personalized shopping experiences powered by AI that knows what you want before you do. It's like having a personal shopper, but it's an algorithm.
AI Ethics and Consumer Trust
This is a big one. As AI gets more involved, people are starting to wonder about privacy and how their data is being used. Retailers need to be super transparent about this stuff to keep customers happy and trusting. It's not just about following the rules; it's about doing what feels right.
The Impact of AI on Customer Experience
AI is changing the whole shopping game. It's making things more convenient, more personalized, and honestly, just more interesting. Imagine walking into a store and getting recommendations based on your past purchases, or using AR to see how furniture looks in your house before you buy it. It's all about making shopping easier and more enjoyable.
The future of retail isn't just about technology; it's about how we use that technology to create better experiences for everyone. It's about finding the right balance between innovation and ethics, and about making sure that AI is used to help people, not just to sell them stuff.
Here's a quick look at how AI might change things:
AI is set to change retail in a big way, and it's going to be interesting to see how it all plays out.
Wrapping It Up
In the end, using AI to tackle product issues before they blow up is a game changer. Companies can spot problems early, fix them fast, and keep customers happy. This not only cuts down on returns but also builds trust and loyalty. As businesses lean more on AI, they’ll likely see fewer headaches and better sales. It’s clear that the future of retail is all about smart tech helping us make better choices. So, if you’re not on board yet, now’s the time to jump in and see how AI can help your business thrive.
Frequently Asked Questions
How does AI help find product issues early?
AI can look at patterns in data to spot problems before they get big. It checks how products fail and uses that information to fix issues quickly.
What is predictive analytics in AI?
Predictive analytics is a method where AI uses past data to guess future problems. It helps companies prepare for issues before they happen.
How can AI improve quality control?
AI can automatically check the quality of products, making it easier to find mistakes. It helps teams make better decisions based on real data.
What is sentiment analysis?
Sentiment analysis is a way AI examines customer feedback to see how people feel about a product. It helps companies understand what customers like or dislike.
Can AI help reduce product returns?
Yes! AI can find the root causes of why products are returned and suggest solutions to fix those problems before they happen.
What are future trends for AI in retail?
In the future, AI will keep getting better and more advanced. It will help businesses understand customers better and improve their shopping experiences.
Author
Trending Post
Get
Inspiration.
@artificial_intelligence_bloom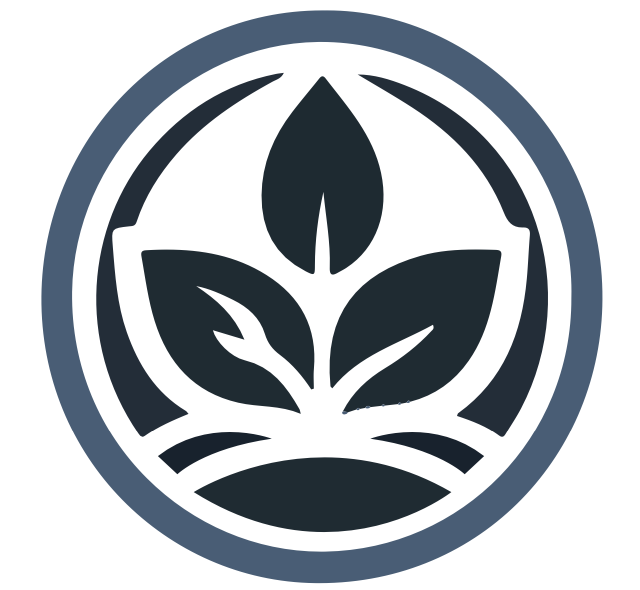